By deploying the algorithm – called TBShoNet – in a smartphone, providers will be better equipped to make a diagnosis on their own.
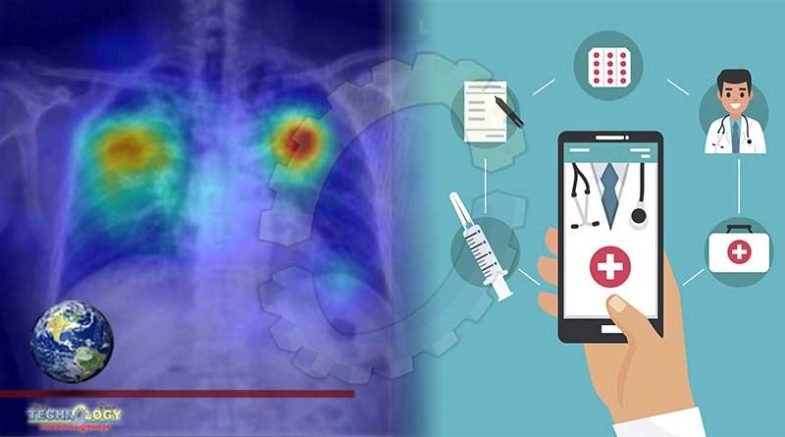
A photo of a chest X-ray captured by a smartphone can be enough for providers to diagnose tuberculosis (TB), according to new research.
In a poster presented during this year’s Radiological Society of North America (RSNA) annual meeting, researchers from National Tsing Hua University in Taiwan explained how using a deep-learning TB detection model with those smartphone pictures can impact diagnosis of the disease. This is the first study to pair a deep learning model with a smartphone for this purpose.
This method is critical, said lead study author Po-Chih Kuo, Ph.D., assistant professor of computer science, because early TB diagnosis has historically been difficult in resource-poor countries where radiologists and high-resolution images are not always available. By deploying the algorithm – called TBShoNet – in a smartphone, providers will be better equipped to make a diagnosis on their own.
“We need to extend the opportunities around medical artificial intelligence to resource-limited settings,” Kuo said.
Kuo’s team used a database of 250,044 chest X-rays with 14 pulmonary labels that did not include TB to pre-train the neural network. They, then, recalibrated the model for chest X-ray photographs, using simulation methods to augment the dataset. Adding an additional two-layer neural network that was trained on augmented chest X-ray images to the pre-trained model completed TBShoNet.
To test TBShoNet, Kuo’s team took 662 chest X-ray photographs – 336 TB and 326 normal – with five different smartphones. According to their analysis, the model produced 81 percent sensitivity and 84 percent specificity for TB classification.
Originally published at diagnostic imaging